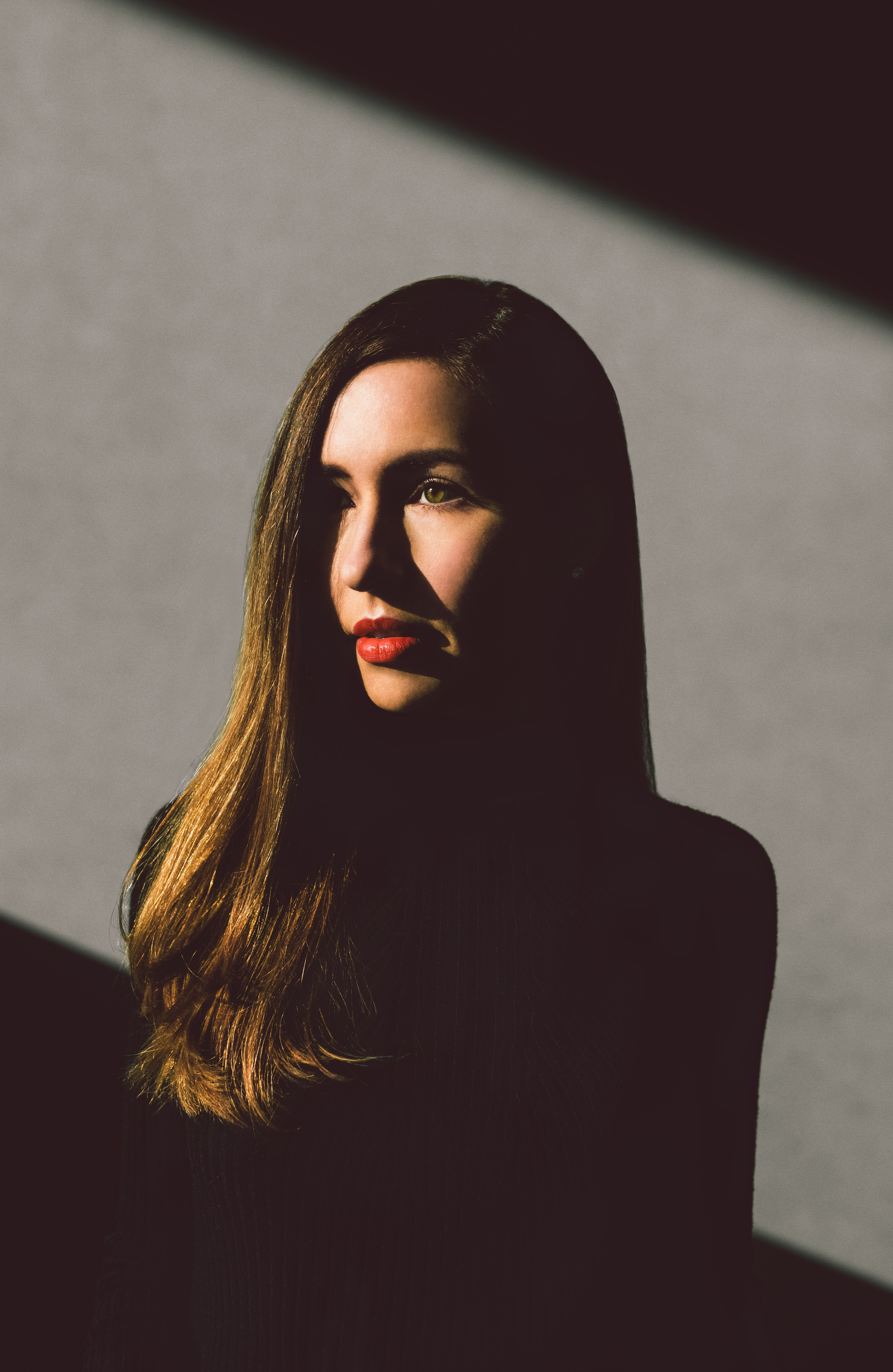
Photo by Chua, Yeong Jiun
Liza Dixon, M.Sc. is a consultant and doctoral candidate researching human-machine interaction in automated driving in partnership with Bosch and Universität Ulm. She coined the term autonowashing to describe the gap between the presentation of driving automation in media & marketing and its actual technical capabilities and human supervision needs.
She has a Master of Science in Usability Engineering from Hochschule Rhein-Waal University of Applied Sciences, where she specialized in advanced driver assistance systems and trust in automation. She is an honors graduate of Flagler College where she received a Bachelor of Arts in Graphic Design, and minored in Psychology and Fine Art.
Her work has been featured by The Next Web, CNBC, PAVE Campaign, The Autonocast, Guidehouse Insights, EETimes, Automotive News and others.
When she’s not thinking about future tech, you’ll find her traveling, cooking with plants, doing yoga or hiking through the woods. She was born in Miami, Florida and now resides in Stuttgart, Germany.
She has a Master of Science in Usability Engineering from Hochschule Rhein-Waal University of Applied Sciences, where she specialized in advanced driver assistance systems and trust in automation. She is an honors graduate of Flagler College where she received a Bachelor of Arts in Graphic Design, and minored in Psychology and Fine Art.
Her work has been featured by The Next Web, CNBC, PAVE Campaign, The Autonocast, Guidehouse Insights, EETimes, Automotive News and others.
When she’s not thinking about future tech, you’ll find her traveling, cooking with plants, doing yoga or hiking through the woods. She was born in Miami, Florida and now resides in Stuttgart, Germany.
Autonowashing
[ ah-ton-uh-wash-ing ]
verb. The practice of making unverified or misleading claims which misrepresent the appropriate level of human supervision required by a partially or semi-autonomous product, service or technology.
Autonowashing makes something appear to be more autonomous than it really is.
Shifting the view of an industry, informing regulatory change, and supporting meaningful progress on road safety
Autonowashing: The Greenwashing of Vehicle Automation
Having identified a gap between scientific literature on human-automation interaction, claims by OEMs, and the reporting on driving automation technologies, an independent journal article was published in 2020. The term autonowashing was coined, and attention was brought to the human factors safety issues and long-term business strategy issue that is making automation appear to be more capable than it is.
To the Publication ︎
Having identified a gap between scientific literature on human-automation interaction, claims by OEMs, and the reporting on driving automation technologies, an independent journal article was published in 2020. The term autonowashing was coined, and attention was brought to the human factors safety issues and long-term business strategy issue that is making automation appear to be more capable than it is.
To the Publication ︎
Automated Vehicles Bill
The Law Commission of England & Wales and the Scottish Law Commission put forth the recommendation of two new criminal offenses for the act of autonowashing. This recommendation was then carried forth in the draft of the Automated Vehicles Bill currently under review by the UK Parliament.
Link to Bill ︎
The Law Commission of England & Wales and the Scottish Law Commission put forth the recommendation of two new criminal offenses for the act of autonowashing. This recommendation was then carried forth in the draft of the Automated Vehicles Bill currently under review by the UK Parliament.
Link to Bill ︎
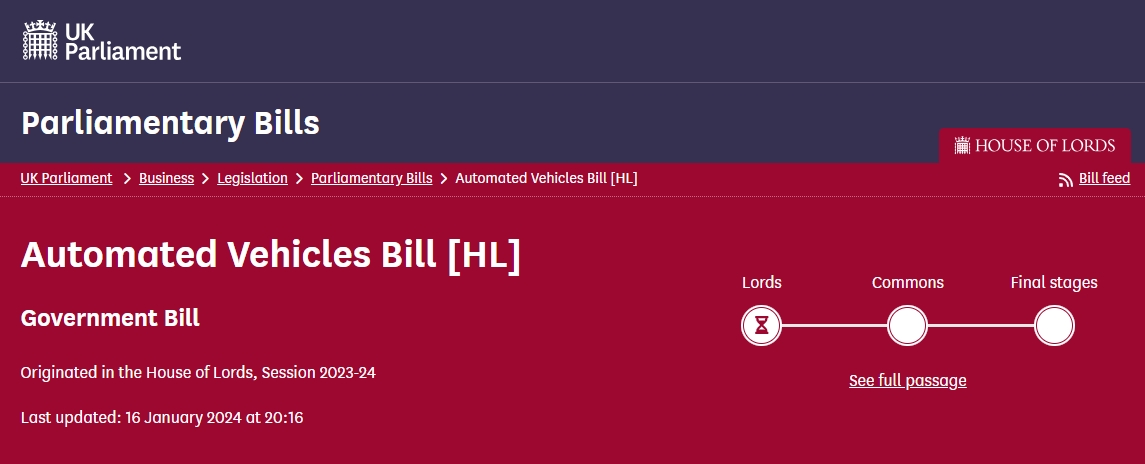
Human-Machine Interaction Design for Authoritative Control Interventions in Driving Automation
Doctoral Dissertation
Highly automated vehicles capable of operating in multiple driving modes and levels of driving automation will mark a significant technological achievement. Further, these vehicles represent a paradigm shift for human-machine interaction (HMI) due to their potential ability to conditionally reallocate control, authority, authority, and responsibility. This may enable them to perform authoritative control interventions in which the automated system fully blocks or fully takes away driver control authority.
Conceptual Development, Experiment Design, Project Management, Data Analysis, Scientific & Technical Communication, Peer-Reviewed Publication
Conceptual Development, Experiment Design, Project Management, Data Analysis, Scientific & Technical Communication, Peer-Reviewed Publication
What might an automated system do when its ability exceeds its human counterpart?
What implications does this have for humans and for HMI design?
Conceptual Framework
To address this paradigm and to lay the foundation for research & development, a historical review of authoritative automation behaviors in the aerospace industry were linked to the driving automation domain. The relationship between regulatory policy, system architecture, Automated Driving System (ADS) mechanisms, and HMI design were explored to support policy discussion. Key intervention types were identified and their interaction patterns visualized.
To address this paradigm and to lay the foundation for research & development, a historical review of authoritative automation behaviors in the aerospace industry were linked to the driving automation domain. The relationship between regulatory policy, system architecture, Automated Driving System (ADS) mechanisms, and HMI design were explored to support policy discussion. Key intervention types were identified and their interaction patterns visualized.
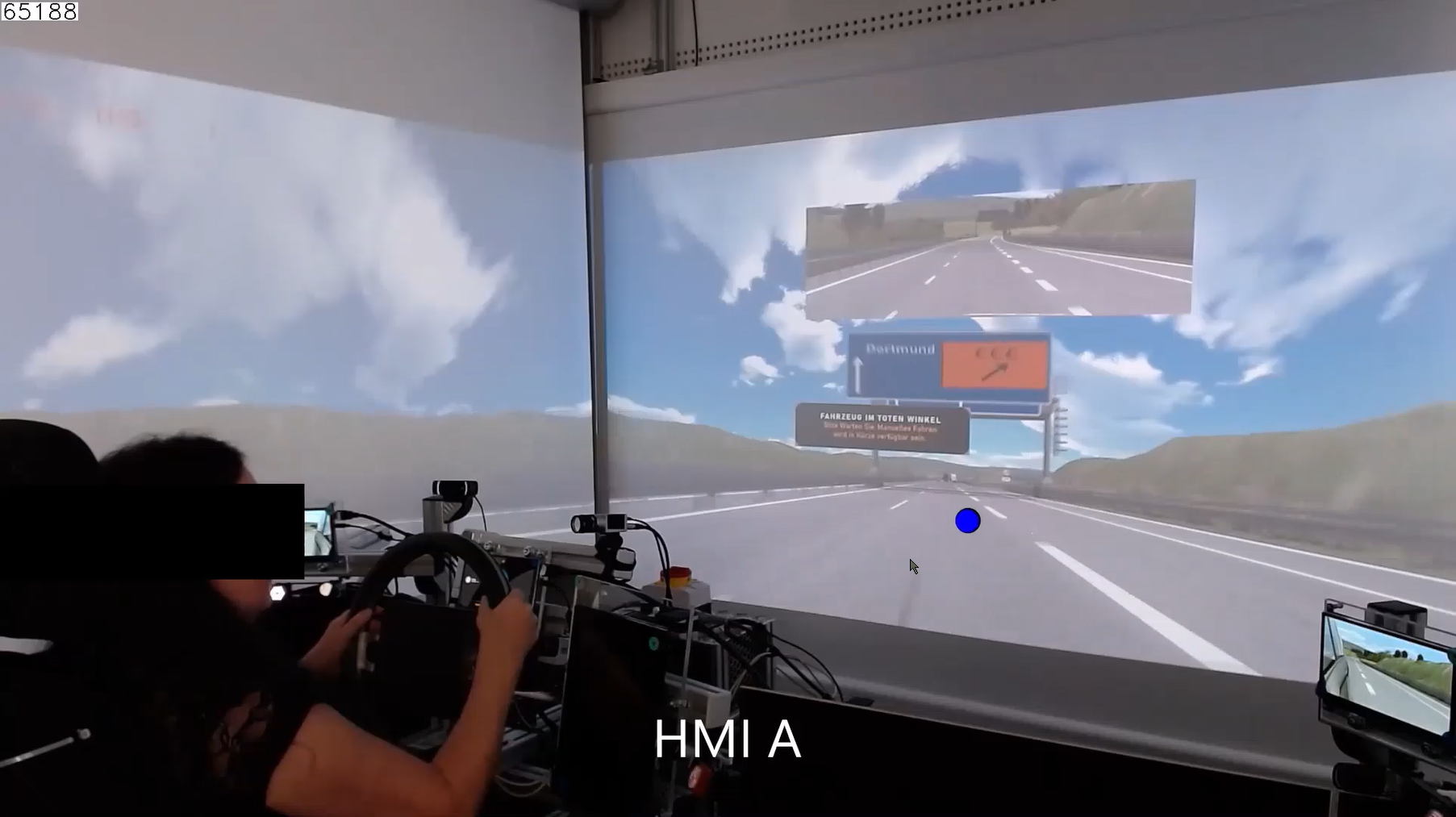
Methods
Multiple surveys and driving simulator studies were proposed, developed, and executed. Throughout the course of study, feedback from a total of over 700 individuals was captured and evaluated to determine driver sentiment towards and interactions with authoritative control interventions. Subjective data gathered from interviews and questionnaires, as well as objective data (e.g., gaze, steering torque) was analyzed to understand how drivers behave when they experience an unexpected loss of control authority.
Experiment Design
A novel incentive concept was developed for the study of authoritative control interventions, enabling study participants to experience spontaneous system interventions initiated by driver control inputs in driving simulator settings.
Multiple surveys and driving simulator studies were proposed, developed, and executed. Throughout the course of study, feedback from a total of over 700 individuals was captured and evaluated to determine driver sentiment towards and interactions with authoritative control interventions. Subjective data gathered from interviews and questionnaires, as well as objective data (e.g., gaze, steering torque) was analyzed to understand how drivers behave when they experience an unexpected loss of control authority.
Experiment Design
A novel incentive concept was developed for the study of authoritative control interventions, enabling study participants to experience spontaneous system interventions initiated by driver control inputs in driving simulator settings.
Results
In simulator, it was observed that drivers express more negative valence at the concept of interventions which block their control input vs. interventions where control authority is removed. In safety-critical scenarios, drivers respond more positively to interventions and express a willingness to give the automation more control. HMI design insights and ethical questions about the development of automated driving are discussed.
Read More ︎
In simulator, it was observed that drivers express more negative valence at the concept of interventions which block their control input vs. interventions where control authority is removed. In safety-critical scenarios, drivers respond more positively to interventions and express a willingness to give the automation more control. HMI design insights and ethical questions about the development of automated driving are discussed.
Read More ︎